AI-Driven Predictive Analytics: New Opportunities for Financial Institutions
AI-Driven Predictive Analytics: New Opportunities for Financial Institutions
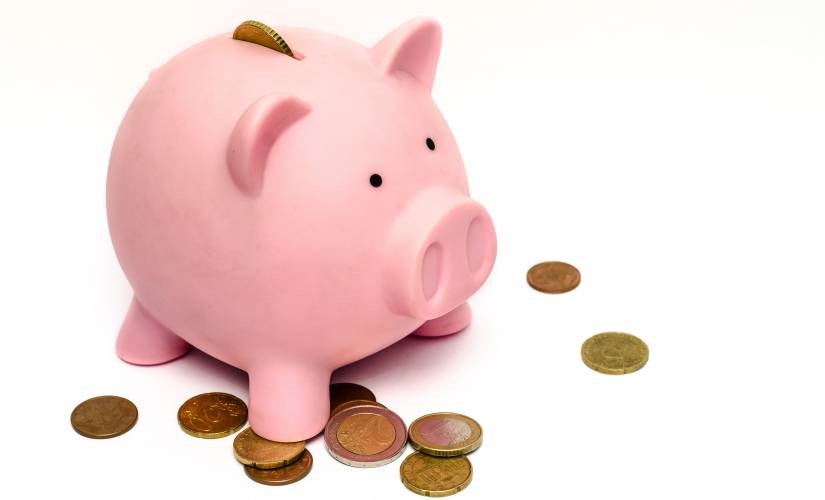
One can argue that even the most innovative banking institutions are bureaucratic enough, and their slow decision-making causing banks to lose their premium over fintech applications, peer to peer lending marketplaces, and payment processors.
We already saw the expansion of mobile app payment services, offering to their customers’ wealth-management products that give higher returns compared to banks.
At the same time, many expanded into the business of micro-lending. Banking services are no longer a monopoly of banks, and traditional financial institutions have to innovate in order to survive.
The era of non-traditional financial services providers such as Amazon Payments, PayPal Payments and PayU, has risen. The launch of the Payment Services Directive II in Europe unlocks new dynamics for FinTech and Payment Services.
The directive facilitates the emergence of common technical standards and supports interoperability within payment providers.
Among the fundamental changes PSD2 brings to the table, is that banks are now obligated to open up their IT infrastructure to Third Party Providers. This regulation constitutes payment services companies as direct competitors of banks.
As identified Account Information Service Providers can now get access to a customer’s account information to aggregate his financial information.
This opens a whole new world of opportunities for consumers to track their spending, optimize their finance, understand better their investment products, control the risk of their investments and more.
Fintech startups and banking institutions conceptualizing a whole new era of products for both retail consumers and enterprise clients. The most popular are: budgeting tools, Robo-advice, AI purchase recommendations, expenditure optimization, savings recommendations, and revolutionary payment ways.
Further to the value of financial products themselves, banks are losing access to customer’s data as they switch to non-banking channels and resources.
Integration with AI
According to a 2017 report by PWC, AI-focused FinTech start-ups have attracted investments with an average of US$1 billion. Until recently, the most common use of Artificial Intelligence in the financial sector has been the operations of virtual customer services — aka Chatbots.
Of course, chatbots are only a small fraction of what AI brings to fintech: robot-trading, anti-money laundering, fraud prevention algorithms, predictive analytics, risk management, credit scores analysis from multiple layers, consumer behavior traction, and customization, are promising to change forever the future of finance.
The potential of insights that could be gained from analyzing the huge amounts of data generated in the Banking and Finance Industry is quite exciting. Of course, if we aim to maximize the performance of AI and Machine Learning algorithm. We need to aggregate data by different sources, argues Quadrant Protocol’s Senior Data Consultant, Glenn Harrison.
The key results from the utilization of AI are the minimization of costs, the swift customer service, automation in the business processes, and most importantly the risk reduction of human error or intentional harm.
Like every new technology, one can wonder if the adoption is for real or there is hype. According to a survey conducted by Financial Times, the current exposure of financial institutions is mainly “narrowed to programs that perform more basic functions that involve logical reason, learning and self-correction without being explicitly programmed, to a vision of AI that includes all automation.”
The same survey warns that “there is a danger that too much investment flows into “hyped” areas such as chatbots at the expense of investment in behind-the-scenes processes where banks could make more significant gains.”
It’s interesting to examine which banks have already implemented AI technologies and examine the level of adoption. JP Morgan is utilizing AI to analyze legal documents and extract critical data points as presented in this video tweet. COin, as they’ve named their AI tool, can proceed within a few seconds 12,000 commercial credit agreements, which would require approximately 360,000 human hours.
Wells Fargo has invested in an AI-drive chatbot which operates through the Facebook messenger. Customers can ask their account balance, the most recent transactions, how much they spent on entertainment last month, and the location of the nearest branch, among others. Bank of America has launched Erica – an intelligent virtual assistant, which serves clients 24/7 and support “day-to-day transactions.”
Bank of NY Mellon Corp. announced they’ve put into force more than 220 bots handling repetitive tasks range from automated programs that respond to data requests from external auditors, to systems that correct data mistakes in requests for funds transfers.
What’s next?
From fully automating manually intensive repetitive tasks, identifying quickly fraudulent transactions, and adopting fast, accurate credit scoring policies AI Technology is expected to shape the future of finance. Accenture’s “Redefine Banking with Artificial Intelligence” report concludes that “AI advances will be so all-encompassing, and so fast-paced, that high-performing organization will inevitably accelerate away and leave the slow movers far behind.”
The transition from chatbots and “virtual customer agents” is bringing a whole new ecosystem with creators building solutions to transform finance. Let’s see who is building and what in AI at the moment.
Endor
Source: Endor World Economic Forum Report ‘Revolutionizing Predictive Analytics for Financial Service”
Endor, which has been called ‘Google for predictive analytics’ is an encrypted data prediction system, that enables predictive analytics while cutting cost & time, providing high-quality results in minutes instead of months. The highlight which positions Endor as a revolutionary innovation, is the ability to offer predictions on encrypted data- without having to decrypt it- which gives a powerful competitive privilege to banks, and no data science expertise is required.
Predictive analytics platforms usually require up to two months to provide the desired results. Endor is using social physics to create a prediction engine which is agnostic to encrypted data – making easy to serve as a model for every prediction. Endor can be used in many domains of banking and financial services to forecast any area of their business: Sales, Marketing, Operations & Risk or Strategy.
SAS AI for Banking
SAS is a trusted analytics powerhouse for organizations seeking immediate value from their data. Across it’s solutions SAS is offering tools from detecting and preventing fraud and financial crimes to managing credit and regulatory risk, to enhancing the customer experience, to generating sufficient capital, SAS solutions with embedded artificial intelligence enhance the speed, precision and effectiveness of human efforts, resulting in a more responsive, more profitable bank.
YITU
Yitu among else is offering an application of facial recognition technology to ATMs in China, in lieu of PIN numbers or fingerprints. Yitu is a Chinese startup that uses artificial intelligence to produce facial recognition data on a wide scale, and has penetrated industries like automotive, healthcare, and are even in the midst of developing chip hardware for AI algorithms.
CAMBRICON
Cambricon is a pioneer in the development of smart chips and processors with high performance, yet low power consumption for AI-related R&D, and have produced a few versions of its AI chip since its launch.
Cambricon Technologies has secured hundreds of millions of dollars in its Series B funding round which sets its valuation at US$2.5 billion, with investments from China’s State-owned Capital Venture, SDIC Venture Capital, China Capital Investment Group, TCL Capital, CITIC Securities, Alibaba VC, Lenovo Capital & Incubator Group and others.
AI is definitely bringing a whole world of new opportunities in the banking sector, with excitement to be translated into investments in AI startups from leading financial institutions.
On the other hand, AI is scaring bank employees which understand the risk that this technology will reduce the need for actual human workers. It is clearly expected that if a bank can fully automate a process through virtual robots, they won’t occupy the expensive time of a human to provide that service.
Non-AI technologies are already digitizing customer services of banking institutions and decreasing the need for in-house staff in banks. The fintech revolution with the rapid expansion of fintech applications is a fundamental threat to the dominance of banking institutions.
Banks offer fast transactions, less expensive, and usually with less hustle compared to traditional banking institutions. Investment in AI is definitely a chance on behalf of the banks to speed up and compete fintech applications.
The post AI-Driven Predictive Analytics: New Opportunities for Financial Institutions appeared first on ReadWrite.
(109)