Data Science Careers: From Fragmentation to Necessity
Data Science Careers: From Fragmentation to Necessity
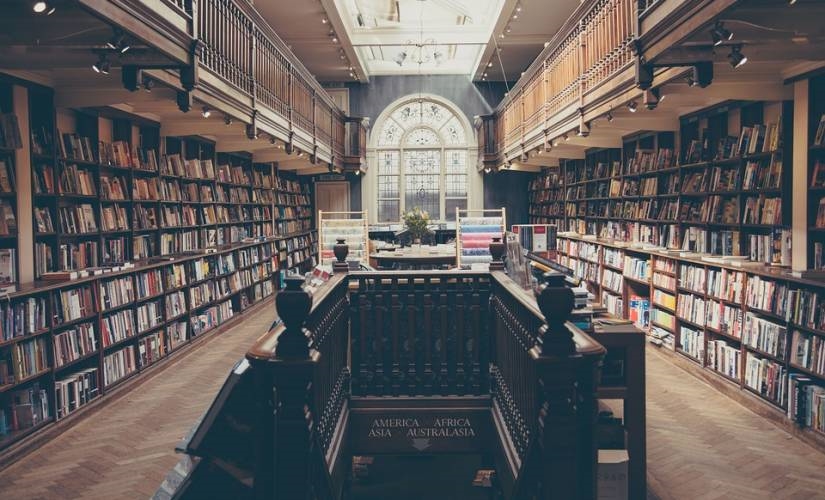
Over the past decade — the concept of being a data scientist has become a “normal” occupation title. It is no longer an unusual way to phrase the job description in data analytics. Now this to the industry standard term for a swath of data careers.
Data Science.
Like any career going through a period of discovery and change, data science is both proving itself vital to emerging markets while also working through misconceptions of what the field entails.
Considering its prominence in machine learning.
Data scientists are also emerging in other tech fields. It’s an interesting career path to follow with more jobs under its umbrella term than might be immediately apparent.
The basics and the misconceptions
As a term — data science refers to what previously may have been known as advanced analytics, econometrics, statistics or machine learning. These jobs all contain a focus on the collection and analyzation of data.
It’s easy to assume a job of this nature focuses on modeling and presenting data.
A large portion of data science revolves around the collection of raw data — and employing algorithms to make the data more easily accessible to humans.
It’s one of many small misconceptions of the field and for good reason.
Industry professionals have described the field as an activity rather than a job title as it covers so many possible routes of employment and individual tasks. Generally speaking, those in the field work with teams of similar skill sets and interests.
Each subset of the field has a different employment of methodologies. These areas of individual expertise are making each scientist very different from the next.
Though some portions of the job descriptor may sound like a data analyst.
The core of data science revolves around applying the scientific method to solving business problems with a heavier focus on mathematics and computing.
Jobs in the data scientist field.
A large number of job postings by potential employers show a preference for data scientists with experience in SQL, Python and Java. Expecting long hours of coding that requires a deep understanding of the math behind different formulae.
Those with experience in the field have pointed out that there are no secret formulae that solve complex business issues. Instead the only methods to finding answers through stringent research and trial-and-error methodology.
Rigorous activity in research.
The upside to all of this rigorous activity? High salary and job satisfaction, according to Glassdoor’s ranked list of the top 50 jobs in America for 2018. These jobs are ranked by potential salary, job satisfaction and the number of open positions posted through the previous year.
The data science field is a fast-growing field that shows almost ten times as many workers in the field as compared to five years ago. Education requirements can be stringent and the field is competitive to the point where having a Master of Data Science might be necessary to stand out amongst competition.
A day in the life of a data scientist
Unsurprisingly, no two experts in the field seem to have an identical itinerary when it comes to their daily tasks. Routines vary wildly and some report not having a daily routine at all. Some researchers instead follow the shifting needs of their workplace.
It’s a field that supports both pre-planners and free spirits as long as they have an interest in problem-solving.
Scientists who responded to the prompt seem to all report a strong focus on communication. Most are backing up the field’s focus on working with teams. Starting off the average workday with a meeting to either set weekly goals or follow up on how those goals are being met seems to be a common thread.
At the same time, updating team leaders and senior management on the progress of these projects. In relatable terms seems equally important, meaning those who have trouble sharing ideas may struggle with certain aspects of the field.
One responder noted he spends roughly eighty percent of his time cleaning data.
This comment is suggesting those periods of communication can be blissfully brief and infrequent — if you find the right workplace.
All of this could diversity could change depending on which field of data science interests you the most. The average day for a machine learning scientist will likely play out very differently than a workday for a data engineer. However, these two fields often work in tandem on similar projects or within the same company.
Looking into the future of the field
If you’re concerned for the future of data science positions — the good news is that the field has existed for some time. Often called and used under many names — even in most cities.
This job title that barely existed as of 2011.
Who would guess at it’s rise to such prominence and near-global use? The data science field is important enough that it is developing clear terminology and has proven itself important.
Looking into the future of all technologies.
Data used in this field is a difficult proposition. Focusing on how the field will continue to prove its merit and become more mainstream has been tackled before — and proves much simpler to hypothesize.
Anthony Goldbloom of Kaggle shared how the O’Reilly’s Strata Conference in 2011 helped bring the field together. Goldbloom spoke in an effort to unify professionals across the industry in a way that proved the cross-compatibility of their skillsets. This at a time when the field was fragmented by non-standard terminology.
There has been a movement to use the concept of applying the scientific method to analyzing data and making business decisions.
This use of the same concept and verbiage has entered the corporate mainstream to the point where many Silicon Valley companies rely heavily on data science. Almost every major decision of a company is now being analyzed by a data scientist.
Enter machine learning.
Machine learning and data analytics have entered the business lexicon quickly and efficiently. This field is bringing businesses away from an era of Excel spreadsheets and idea-pitching meetings. Predictive data models and experts solve problems are being solved before they even arise.
As the field advances, reliance on this style of predictive problem solving can only increase — making for a stable field of employment for those with the right aptitudes.
For those who enjoy breaking down problems through rigorous testing will find fulfillment in solving business problems.
At the cutting edge of their field — data science is a tempting job title to consider chasing. It holds the promise of longevity with room for developing new skills and honing old ones. This field holds the edge of being able to share your solutions with those people and businesses who need them most.
In short, it’s a problem-solver’s dream.
Tags: No Tags Found
(100)