Did You Hear That? Robots Are Learning The Subtle Sounds Of Mechanical Breakdown
Sometimes, machines just don’t sound right.
Brakes squeal, hard drives crunch, air conditioners rattle, and their owners know it’s time for a service call. But some of the most valuable machinery in the world often operates with nobody around to hear the mechanical breakdowns, from the chillers and pumps that drive big-building climate control systems to the massive turbines at hydroelectric power plants.
That’s why a number of startups are working to train computers to pick up on changes in the sounds, vibrations, heat emissions, and other signals that machines give off as they’re working or failing. The hope is that the computers can catch mechanical failures before they happen, saving on repair costs and reducing downtime.
“We’re developing an expert mechanic’s brain that identifies exactly what is happening to a machine by the way that it sounds,” says Amnon Shenfeld, founder and CEO of 3DSignals, a startup based in Kfar Saba, Israel, that is using machine learning to train computers to listen to machinery and diagnose problems at facilities like hydroelectric plants and steel mills.
And while most current efforts are currently focused on large-scale machinery, Shenfeld says the same sort of technology might one day help detect failures in home appliances or in devices like self-driving cars or rental vehicles that don’t spend much time in the hands of an owner who’s used to their usual sounds.
“When you have car-as-a-service, the person in the car doesn’t know the car,” he says. “If it sounds strange, you’re losing this link with the human in the car deciding it’s time to take it to the mechanic.”
Initially, 3DSignals’ systems can detect anomalous sounds based on physical modeling of particular types of equipment, notifying an expert mechanic to diagnose the problem. And once the problem is fixed, the mechanic’s diagnosis is added to 3DSignals’ database, which it uses to train its algorithms to not only detect unusual sounds, but also interpret them to understand what kind of repair is needed.
“The next time we hit this signature on the same machine for the same customer or another customer using the same type of machine, it will not just be anomaly detection,” says Shenfeld.
And while 3DSignals focuses entirely on using its machine learning tools to process acoustic data—an area Shenfeld says is surprisingly neglected outside of speech recognition—other companies are using a variety of other types of data to detect and diagnose mechanical problems.
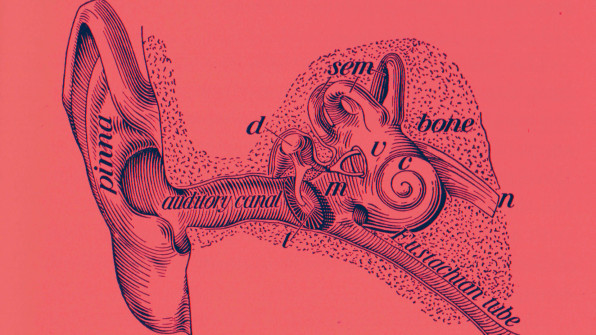
Systems from Augury, a startup with offices in New York and Haifa, Israel, monitor vibrations, temperature ultrasound, and electromagnetic emissions from machinery, typically large-scale heating and cooling systems. The company offers a portable tool a technician can use to capture a reading to an iPhone or Android device, where an app offers a real-time diagnosis as well as a continuous monitoring system, says cofounder and CEO Saar Yoskovitz.
“One of our customers, located in Ohio, had a thunderstorm at 2 a.m., and the whole building went dark,” he says. “The generator was supposed to pick up automatically but it didn’t, and the way that they learned about it was through an alert they got from Augury.”
In more complicated situations, the system uses machine learning-based algorithms and a growing library of signals of different types of errors to suggest what’s wrong and what technicians can do to repair the machine, he says.
“Over time, we’ve collected probably the largest malfunction dictionary in the world for our types of machines,” says Yoskovitz.
For another startup, called Presenso, also based in Haifa, the exact type of data being monitored is less important than how it changes over time and what that signals about the machine’s operation. The company’s systems record data from sensors already installed in industrial equipment as part of existing control processes, streaming readings to Presenso’s cloud servers.
“We don’t care, or we don’t need to know, if the sensor we’re analyzing now measures temperature or voltage or flow,” says CEO and cofounder Eitan Vesely.
Presenso’s software tools use a variety of machine learning techniques to effectively build a model of a machine’s operations based on the sensor data it receives. The tools provide visualizations of unusual readings, and how different they are from normal operations.
“They don’t need any human guidance or [to] know what the physical attributes are that are being measured,” Vesely says. “The goal is for them to learn by themselves how the machine operates.”
And while real-time breakdown detection is obviously useful for companies operating machines in their own facilities, experts say it could also be useful to equipment vendors or insurance companies providing financial coverage in the case of downtime.
“If a company has downtime or business interruption insurance, that’s something where it becomes incredibly relevant and also a money saver,” says Ryan Martin, a senior analyst at ABI Research.
Having more reliable predictions of when machines need maintenance could also spur equipment makers to effectively offer time as a service, charging industrial users either extended warranties or even charging by the hour for the guaranteed use of their equipment without incurring too much risk to themselves, says Augury’s Yoskovitz.
“One of the problems with this business model is they hold all the risk,” he says. “If anything goes wrong, they pay for it, and they’re looking for ways to lower this risk.”
Fast Company , Read Full Story
(34)