Facebook’s Formula For Becoming The Athens Of AI
Facebook is known for a variety of mantras embedded in its culture, often spelled out on signs at its offices or recited by CEO Mark Zuckerberg and other executives: “Code wins arguments,” “Move fast and break things,” or “Done is better than perfect.”
A sign on the wall at the company’s New York office perfectly sums up the approach Yann LeCun brings to his leadership of Facebook’s nascent efforts in the field of artificial intelligence and machine learning: “Always be Open.” Artificial intelligence has become a vital part of scaling Facebook. It’s already being used to recognize the faces of your friends in photographs, and curate your newsfeed. DeepText, an engine for reading text that was unveiled last week, can understand “with near-human accuracy” the content in thousands of posts per second, in more than 20 different languages. Soon, the text will be translated into a dozen different languages, automatically. Facebook is working on recognizing your voice and identifying people inside of videos so that you can fast forward to the moment when your friend walks into view.
Facebook wants to dominate in AI and machine learning, just as it already does in social networking and instant messaging. The company has hired more than 150 people devoted solely to the field, and says it’s tripled its investment in processing power for researchâthough it won’t say how much that investment is.
If the mobile cloud was the previous era of computing, the next will be the era of AI, says Jen-Hsun Huang, the CEO of Nvidia, one of the worldâs largest makers of graphics processors and a partner in Facebookâs open-source hardware design. “It is the most important computing development in the last 20 years, and Facebook and others are going to have to race to make sure that AIâs a core competency.”
Yet Facebook, which only seriously entered the field less than three years ago, will need more than money to compete, since it’s one of technologyâs hottest fields right now. “They were a late comer,” says Pedro Domingos, a professor of computer science at the University of Washington and the author of The Master Algorithm. “Companies like Google and Microsoft were far ahead.” They’ve been building intelligent software since well before Mark Zuckerberg announced plans to program an intelligent butler that would control his home.
Microsoft, which has been working on machine learning since 1991, has several hundred scientists and engineers in dozens of research areas related to the field. Google Assistant, the centerpiece of that company’s deep learning efforts, is on the way to becoming the front-end brain for most of its apps and services. Chinese search giant Baidu poached the head of Google’s deep learning project, Andrew Ng, back in 2014. OpenAI, a nonprofit, has $1 billion in funding from Tesla founder Elon Musk and other tech heavyweights. Amazon CEO Jeff Bezos, speaking at the Code conference, said his company has been working on AI behind the scenes for four years and that it already has a thousand people dedicated to its voice recognition ecosystem. Apple and Uber have also invested heavily in artificial intelligence, and are competing to attract the same pool of talent.
All of this is riding on a wave of striking innovation in the field, some of which came from LeCun himselfâwidely considered one of the most accomplished scientists in the fieldâduring his pre-Facebook days. And Facebook has rapidly gone from not having a formal research lab of any kind to housing two of them. Facebookâs Artificial Intelligence Research program (FAIR), headed by LeCun, focuses on fundamental science and long-term research. Then thereâs the Applied Machine Learning (AML) division, led by Spanish-born Joaquin Candela, a longtime machine learning expert who, among other things, created a course on the topic at the University of Cambridge. His team finds ways to apply the science to existing Facebook products.
The two divisions are separate, with both LeCun and Candela reporting to Facebook CTO Mike Schroepfer. The challenge is figuring out how to make the two groups work together, with long-range scientific research feeding into near-term business goals. One obvious way to make that happen: Get the two teams sitting next to each other. “They have to have personal relationships,” says LeCun. “And they have to collaborate really closely.”
At Facebook, they not only sit next to one another but near the very top of the organizationâjust feet from Zuckerberg’s and Schroepfer’s offices, in factâa sign of how valuable AI and machine learning has become to the company.
But just because you sit next to someone doesn’t make the task of capitalizing on deep science any easier. To understand how LeCun and Candela plan to make it work, you have to first understand where LeCun and Candela came from.
Facebookâs Artificial Intelligence Research Lab
Thereâs a big blue thumbs-up logo taped to the front door of Yann LeCunâs office in the computer science department at New York University. LeCun, one of the worldâs foremost experts in deep learning, didnât put it there. Wearing a navy blue polo shirt with a small image of Einstein stitched above the word “THINK” on a recent Wednesday, he laughs and says that when it was announced two and a half years ago that he was joining Facebook, someone put it there, and he just never took it down.
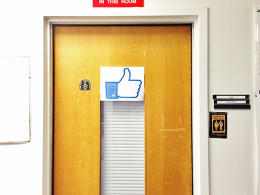
LeCun, 55, is still a part-time professor of computer science at NYU, which is located just steps from Facebookâs posh Big Apple digs. Youâd never pick him out of a crowd as the one spearheading the massive AI ambitions of the worldâs largest social networking companyâyet heâs also the kind of guy whose first ride in a Tesla sedan was with Elon Musk.
If you’ve ever deposited a check using an ATM, then you’ve probably seen LeCun’s research at work. As one of the fathers of a branch of deep learning known as convolutional neural nets, LeCun is a celebrity in the world of AI. Thatâs because ConvNets, as theyâre sometimes called, are today considered the building blocks for developing scalable automated natural language understanding and image recognition tools, and even voice recognition or visual search systems, all of which are immensely valuable to Facebook, Google, Baidu, Microsoft, and others. LeCunâs work in the field focused on models that aimed to replicate the way living beingsâ visual cortexes work.
LeCun was given broad freedom to build FAIR as he saw fit, adding people and bringing structure to a group of about a dozen AI researchers in the U.S. that pre-dated him. There was plenty of rationale for Zuckerberg and Schroepfer to grant LeCun that freedom: Heâd spent 14 years at Bell Labs and developed a sense for what worked, and what didnât, and had been thinking all along about how he would set up a new research lab if given the chance.
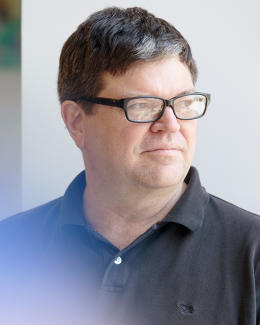
The key to success, he believes, is a dedication to openness. LeCun’s dual lives in industry and academia are grounded in a philosophy dictating that researchers publish their work for all to see, speak at conferences, interact widely with academia, and post code to open-source repositories like GitHub.
“Iâve seen lots of my friends join [big tech companies] coming from research labs that had a culture of openness and try to change the culture of the company and completely fail,” says LeCun. One of the first questions he asked before joining Facebook was about its commitment to the open-source world and a culture of openness.
He also wanted to nail the balance between doing research and translating that work to product. Many tech companies, he felt, had trouble figuring out how to do that without the researchers losing their focus. Perhaps the most notorious example is the work done by Silicon Valleyâs legendary Xerox PARC on the graphical user interface, which Apple applied to the Lisa, and then the Macintosh, after Steve Jobsâs famous visit in 1979.
One model LeCun had seen fail was called “hybrid research,” where scientists are embedded in engineering groups. That usually stunted their creativity. Another involved hiding researchers away in an ivory tower with little communication with the rest of the company. That was good for stature, but little else.
LeCun would know. From 2002 to 2003, he worked in NECâs prestige lab at Princeton, an advanced research shop the Japanese company had set up with no real urgency to impact product. “They never asked them to produce anything for the company,” he says, “Then all of a sudden they did. They told these people it would be nice if you produced stuff we could use, and basically everybody left. Including me, by the way. And it was impossible to break the barriers that existed between research and development.”
Under LeCunâs direction, FAIR launched in December 2013 with a focus on long-term problems in artificial intelligence and machine learning. Facebook knew that to achieve both the short-term and long-term benefits of his team’s work, it had to have some scientists and engineers that work on developing new techniques that will impact the field years from now, while others focus on impacting current product. Perhaps 70% of the groupâs work is research, LeCun estimates, while 30% is near-term development.
“We are more outward focused,” LeCun explains, “so we publish a lot of things we do [and] distribute a lot of code on open-source. So weâre really part of the research community, because we really want to push the envelope, push the technology forward, push the science forward. And make sure we have the expertise and have control of the best state-of-the-art technology of the moment, and we kind of drive the progress in that direction.”
The groupâs goals are ambitious: teaching machines common senseâin essence giving them the ability to learn the way a baby or an animal does, for example. FAIR’s biggest project right now, LeCun says, is natural language understanding for dialogue systems, which will be the basis of Facebookâs intelligent voice assistants.
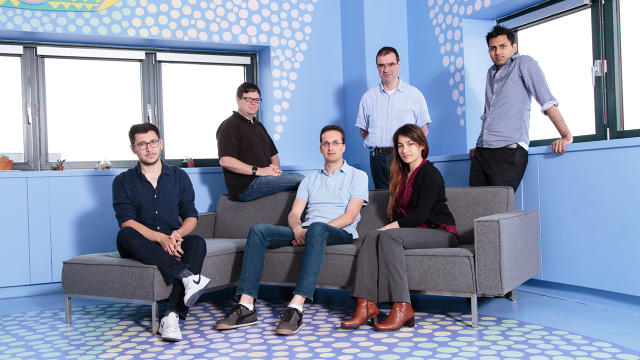
Itâs already evident that every major tech company wants to be the leader when it comes to voice assistants. The most famous example is Appleâs Siri. But Microsoft is in the game with Cortana, Amazon has its Alexa, and then thereâs Viv, the brand-new project from the team that built the pre-Apple Siri at SRL International.
Facebook has its own plans for intelligent voice assistants, like its year-old effort, M. And AI is at the heart of it because in order for a system to actually make a difference to users by successfully answering just about any question, it has to have common sense, LeCun argues.
“That means, how do we get machines to learn just by observing the world,” he says, “as opposed to being trained to [explicitly] recognize tissue paper, cars, cell phones” and other things.
Today, the technology doesnât exist to give machines common sense. The solution, LeCun believes, isnât to solve the problem by attacking it directly. Instead, you have to figure out how to get machines to understand text, and that in turn means teaching machines enough background knowledge about the world that they can understand it.
“If I say, âThe trophy didnât fit in the suitcase because it was too small,â you know that the âitâ refers to the suitcase, not the trophy because you know what it means to put something into something else.”
A machine doesnât understand that, and getting to that level of understanding is one of FAIRâs long-term goals.
Achieving the ability to have that sophisticated common sense and text understanding would impact not just voice assistants but also automatic language translation, a feature Facebook considers crucial as its user base grows internationally.
“Translation is a hugely important thing,” LeCun says. “The main mission of Facebook is connecting people, and the first thing you have to do is make sure the communication works between people through translation.”
But first, he’ll need to make sure that the work his team is doing at FAIR gets translated and communicated to the people sitting just a few feet away.
The Applied Machine Learning Lab
Sitting in Facebook’s Frank Gehry-designed headquarters, Applied Machine Learning chief Joaquin Candela is dwarfed by a massive box overflowing with giant stuffed animals. Unfazed when no conference room was available, the bespectacled 39-year-old didn’t blink at the notion of taking the conversation to a pair of forgotten couches in a dark, abandoned corner of the otherwise bustling, light-filled building. When it was time to rush off to a meeting with Schroepfer, Candela graciously offered to carry a reporter’s recorder and keep talking during the long walk to the CTO’s office.
LeCun was already at Facebook when AML was hatched, indeed he pushed for it to be created, “because I thought it would be the primary channel through which technology developed at FAIR would make it into products.”
AMLâs goal is “to advance the state of the art for maximum product impact” and to be “the glue between science and research and product impact.” Developing better algorithms for ranking feeds, ads, and searches, language translation, speech recognition, generating automatic captions for videos, and natural language understanding are all areas in which AML is trying to actively improve Facebook’s bottom line.
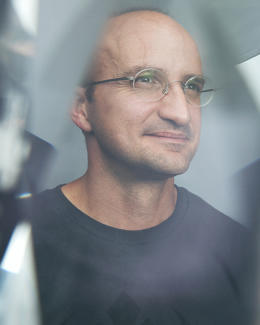
When he was asked to start AML, Candelaâwho had been a Facebook engineering manager running a team that built machine learning infrastructureâwanted to avoid the mistakes he’d seen other applied research labs make. “I’ve just seen things done that led to sub-optimal transfer of science to engineering,” says Candela, a veteran of five years at Microsoft Research and Germanyâs famous Max Planck Institute.
That included the mistake of having the labs be too disconnected from engineering, or indulging a culture where the researchers aren’t focused on goals connected to impacting product.
Whereas LeCun’s group spends 70% of its time on research, the reverse is true for Candela’s team, where the majority of time is spent on applying the research to deployable products. Candela says his group thinks about projects in terms of quarters or monthsârather than the five to 10 year time scale that LeCun’s team works onâand generally does its planning in six-month chunks, even though much of what the group works on is “guided to where we want to be two years from now.”
Despite the differences in what they are working on, both Candela and LeCun agree that a dedication to openness will lead them to greater success. CTO Schroepfer agrees, and is quick to quantify some of the ways the company has practiced the philosophy. Along with open-sourcing its hardware and data centers, he says Facebook engineers have published more than 10 million lines of open-source code, and that that there are 350 active GitHub projects in production.
That kind of openness has become essential for hiring great people. “Where do top scientists want to go and work?” Candela asks. “Well, they want to go and work with like-minded people, and how can they know if we have those like-minded people? Well, because you see what they’re working on. You see what they’re publishing. You understand the problems they’re trying to solve, and how they’re trying to solve them.”
One of AMLâs newest teams is computational photography, formed when Rick Szeliski and several others jumped ship from Microsoft Research last October. That team will focus on things like stabilizing videos, including 360-degree videos, helping people take better selfies, and organizing visual content on their phones.
“We came to Facebook because this is where the photos are, and where the data is,” says Szeliski, who led Microsoft Researchâs Interactive Visual Media group. “Itâs this big warehouse of stuff we can analyze. We can touch pixels every day, and delight users, and make them happier and more likely to take more photos and share more stuff. So itâs not just where the photos are, itâs where the photos flow.”
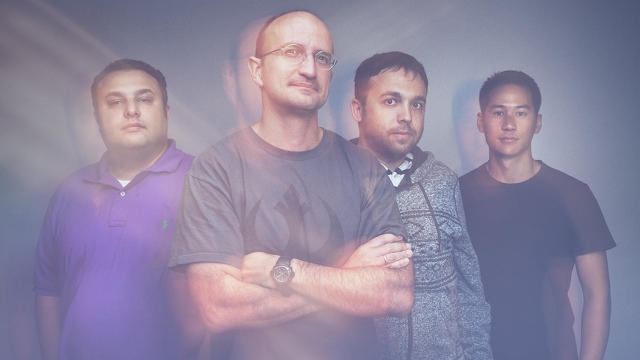
“Flow” is a word heard often at Facebook. Partly in reference to FBLearnerFlow, an end-to-end research and engineering pipeline created by AML that is something of a killer app for testing and sharing machine learning, albeit one that for the moment at least, is only used internally. Itâs a repository where anyone at Facebook focusing on AI or machine learning can post their work for other engineers to use in their own projects.
“Imagine we have a new ads vertical, doing rich ads that go into Instant Articles, and that team just doesnât have a lot of machine learning expertise,” the genial Candela told me in his thick Spanish accent. “So [those engineers] will actually be able to go to [Flow] and browse every single experiment and production model that is running across the entire company, be able to take modules [and use them for their own purposes]. I always encourage people to beg, borrow, and steal. You donât have to reinvent the wheel.”
Flow is also a platform for testing new features in a controlled environment. “This is the beautiful thing,” Candela says. “It’s one place that takes you from research all the way to live experiments, and then if we’re doing an experiment that 1% of people interact with, and it looks good, then we start rolling it out to 100% of the people.”
That broad utility is why Flow is already being used by a quarter of Facebookâs engineers, not just the ones in AI.
Flow is also a perfect representation of how Facebook practices openness internally: Instead of siloing research, it’s made available for all to see.
Facebook’s dual long-term and short-term research dynamic has earned it some respect in the A.I. and machine learning communities, but that’s no guarantee it will manage to succeed at fulfilling the kinds of 10-year visions loudly touted by Zuckerberg, Schroepfer, and company.
There are plenty of ways it could fail, privacy issues being the most obvious one. As Facebook users realize the extent to which the company is analyzing their every post, their every photo, and burrowing its way further and further into their lives, they could begin to push back.
Thereâs also a financial question: At what point will Facebook’s top management and board demand to see a return on its AI spending? Schroepfer insists that Facebookâs management isnât worried about FAIR or AMLâs return on investmentâ”I think both groups have paid for themselves for the next 5 or 10 years easily,” he said. “We donât bother to calculate the ROI on it, because one or two projects, it was like, yup, that probably did it.”
But given how much emphasis everyone in leadership positionsâLeCun, Candela, Schroepfer, and othersâputs in the essential nature of being open, what happens if conditions change that convince those leaders to back off on the philosophy?
“If they move away from this, like many companies have in the past, they might lose their edge in research,” said Yoshua Bengio, head of the Machine Learning Laboratory at the University of Montreal and a former Bell Labs colleague of LeCun’s. “There is a natural tendency in companies at some point, maybe when things arenât as rosy, to push researchers toward delivering short-term results, which hurts labsâĤand long-term prospects for the company itself.”
The challenge, Bengio adds, is to install strong peopleâlike LeCun, he saysâas buffers between products people and research people, to make sure thereâs not too much pressure to deliver short-term results.
But “itâs going to continue to be a challenge [for Facebook] in the future, because thereâs always pressure for short-term objectives.”
Even LeCun recognizes that the ground can shift without warning.
“Nobody is forcing us to justify our existence, yet,” LeCun said. “But I know for a fact, having lived through several life and deaths of industrial research labs, that unless there is something where you can say, like, here is something we are doing for the company, here is why youâre spending all that money, that only lasts so long.”
It’s for exactly these reasons that LeCun and Candela have been so careful to construct their labs in ways they think maximize the potential for success.
“The more you can be crisply clear on what an organization does, and the fewer things it is,” Schroepfer said, “the better it’s going to to that thing. So if you ask them to do 10 things, they’ll do three of them well, kind of, and the other seven terribly. So you’d better hope they picked the right three. Whereas, if you say this organization is here to do one thing, then you can really see how it’s doing. And we have basically two different problems to solve:” research for the future, and finding ways to apply it to product now.
That means creating effective ways to share ideas across the organization. “The dream scenario, and itâs one weâre working toward,” Candela says, “is one where you can have this circulationâĤ(AML) people who will join FAIR” and vice-versa.
It’s already happening. Candela said Facebook’s facial recognition team was started at FAIR, and then moved to AML as its work became more relevant to product. As did the computer vision team, whose leader, Manohar Paluri, still straddles both groups.
On the other hand, Candela cited the example of a researcher doing work with AML’s machine translation team. He “was passionate about doing research on neural networks, applied to machine translation. And so his focus was on pushing the state of the art and advancing science, and he moved…to FAIR.”
LeCun notes that plenty of infrastructure built by one group flows to the other. For example, the DeepText project the company just unveiled was a direct implementation by AML of work originally done at FAIR on trying to figure out how to classify text and understand text using ConvNets and other deep learning techniques.
When they do make advances like that, Facebook isn’t interested in keeping them locked away where the rest of the research community won’t be able to see them. In the case of DeepText, that’s evidenced by an in-depth, explanatory post on Facebook’s open-source Code blog.
“You get the best of both worlds,” Paluri said. “You’re actually publishing in academia, you’re attending the conferences, and you’re actively contributing to the science. And at the same time, you’re seeing that whatever engineering and science you worked on is impacting billions of people.”
Some might worry that there’s business risk in being open like this, but LeCun scoffs at the notion. Facebook gets the benefit of having outsiders work on their code, because if they’re good, Facebook can hire them. Or the company can simply adopt their improvements.
“It’s okay if other people use our technology because [its value] is rarely just in the technology itself,” LeCun said. “It’s in the way we can exploit it because of our position in the market. And we’re pretty big at the social network business. And so if we invent a technology that can be applied to this, we’re going to be the ones to take advantage of it the fastest.”
On the other hand, he added, “If we don’t take advantage of it before other people, it’s our own fault.”
See where Facebookâs internet manifest destiny beganâand how the site got to where it is today.
Fast Company , Read Full Story
(78)