Machine Learning and Exception Management – A Logistics Tech Game-Changer
Machine Learning and Exception Management – A Logistics Tech Game-Changer
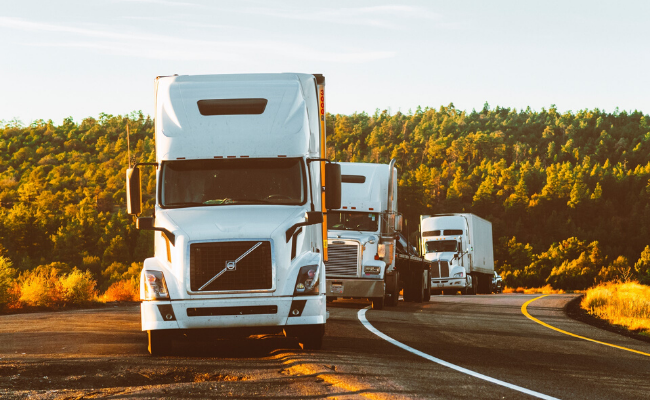
There has been a lot of talk about machine learning in logistics management. The idea is simple: optimize, infer, implement and repeat. Here is: machine learning and exception management — a logistics tech game-changer.
What is included in the different pillars of logistics management?
A system optimizes the different pillars of logistics management that include order planning; vendor performance management; fleet capacity optimization (management); dispatch management; in-transit shipment tracking; and delivery management.
Next, the system infers the points or bottlenecks within these pillars (logistical processes) which can be fixed, improved, or enhanced. These inferences or analytics are then ‘implemented’ back into the logistics set-up. The learning mechanics start back from optimization. Over-time the system evolves and improves all the connected logistics management processes. This is machine learning in logistics management.
What is exception management in logistics?
A logistics exception (issue) is a deviation from planned or expected process execution. Here are a few examples.
- Shipment loads aren’t mapped properly to available fleet options (creating capacity-mismatches and loading/dispatch delays).
- In-transit shipments are detained at a spot for more than two hours (or are violating service level agreements with speeding or harsh braking).
- Consignees didn’t receive all the SKUs (stock-keeping units) as per the initial purchase order.
Every transportation management system (TMS) involves some or many human touchpoints. A person supervises these system or process interactions (touchpoints). This can be anything from checking the shipment assignment schedule and ensuring that the handlers are following the planned loading patterns. Similarly, many other touchpoints work to ensure that the gap between plans and ‘actuals’ is minimal.
The goal of exception management is to minimize this gap between planned and on-ground results. Overall, the machine-learning aspect of exception management induces accountability and efficiency within the company’s and logistics network’s culture. This can be with the supervisors, warehouses, freight forwarders, logistics service providers, consignees (distribution points), etc.
6-stages of machine-learning enabled exception management system.
The 6 stages are Discovery, Analysis, Assignment, Resolution, Records, and Escalation.
Discovery:
It detects and reports issues or anomalies within the processes. This can be through temperature sensors (cold-chain logistics), real-time movement tracking, order journey tracking (in-scan and out-scan of each SKU), etc.
Analysis:
It analyses and processes the issue or exception as per protocols (or learnings). It categorizes and pushes ahead all exceptions – either to an assignment or to an escalation.
Assignment:
It matches the exception with the right person or department (best-suited to resolve the exception on time).
Resolution:
It tracks the speed and effectiveness of the person’s (assignee) resolution. It moves the ‘resolution’ through multiple criteria and validations before satisfactory ‘completion’.
Records:
It records and analyses each exception right from discovery to resolution. The system processes these records to throw-up insights or best-practices for future applications.
Escalation:
This is an important aspect of dynamic exception management. The system constantly tracks each issue within the system.
- If at the analysis or resolution stage, the supervisor (or system) deems the issue – critical or complicated, then it’s escalated through special ‘analysis’ and resolution. It mostly includes people with different skill-sets or authority.
- If the system detects that an issue hasn’t been resolved in its time-frame, it’s again escalated.
Through these 6-stages, the system constantly weeds-out inefficiencies from within itself. It helps propagate a more transparent, accountable, agile, and responsive culture. Furthermore, it helps reduce errors and delays, which, in turn, improves profit margins. A few new-age TMS start-ups, like Fretron, are trying to capture market share using this 6-stage exception management.
Real-world applications of escalation management in logistics
Let’s consider a real-life use-case for an exception management system (EMS) – a fast-growing retailer in India focusing on Tier-2 and Tier-3 cities.
Their biggest challenge was an unorganized logistics (vendor/freight forwarder) network and weak city infrastructure. Even though the retailer had opted-in for total logistics automation, they still weren’t able to implement it to the full extent. The client was looking for a tech-enabled process and culture change.
Let’s take vendor performance management as an example.
- The EMS helped cut down discrepancies in billing and settlements. A single synchronized TMS was able to track each order (at the SKU level) as it moved through crates, pallets, trucks, cross-dockings, and final delivery. The out-scan could automatically highlight all the missing items.
- The EMS would process the information and mark the exact point of deviation where the item went missing. This helped with issue resolution and also to plug these operational gaps. It cut down invoice-level disputes and hastened the settlements.
- The EMS enabled fast and error-free invoicing which incentivized the carriers and freight forwarders to work in a more organized fashion. Through an iterative learning process, the system improved upon itself. It brought a higher degree of transparency and accountability within the logistics ranks (in the company).
- On the back of machine learning-enabled EMS, the company was able to deliver on-time value (better shelf choices) for its end consumers.
Conclusion: Exception management, in logistics, is a game-changer
EMS successfully bridges the gap between tech-induced efficiency and on-ground employee efficiencies. It’s especially effective in unorganized or traditional markets that are riddled with such ‘exceptions.’
If machine-learning backed EMS is used in the right manner, many mid-level companies can scale fast and improve their outlook within the next five years. At this time of COVID-19, scaling faster may be the only option to save your company.
The post Machine Learning and Exception Management – A Logistics Tech Game-Changer appeared first on ReadWrite.
(20)