NYC bill bans AI recruiting tools that fail bias checks
Facebook asked people to share their age and gender to create a fairer AI dataset
Properly labelled information can go a long way toward combating bias in AI.

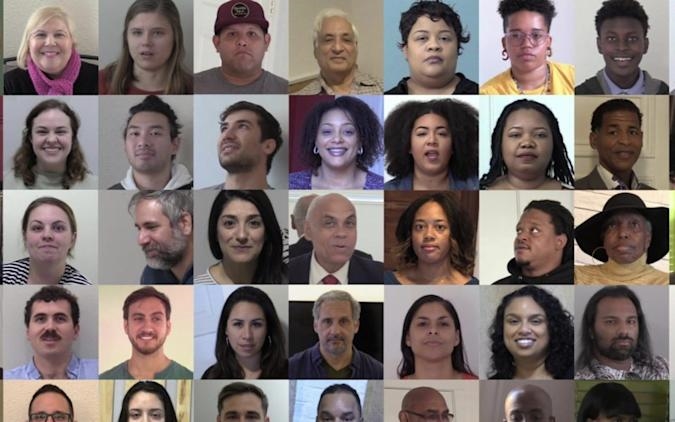
Facebook is sharing a new and diverse dataset with the wider AI community. In an announcement spotted by VentureBeat, the company says it envisions researchers using the collection, dubbed Casual Conversations, to test their machine learning models for bias. The dataset includes 3,011 people across 45,186 videos and gets its name from the fact it features those individuals providing unscripted answers to the company’s questions.
What’s significant about Casual Conversations is that it involves paid actors who Facebook explicitly asked to share their age and gender. The company also hired trained professionals to label ambient lighting and the skin tones of those involved according to the Fitzpatrick scale, a dermatologist-developed system for classifying human skin colors. Facebook claims the dataset is the first of its kind.
You don’t have to look far to find examples of bias in artificial intelligence. One recent study found that facial recognition and analysis programs like Face++ will rate the faces of Black men as angrier than their white counterparts, even if both men are smiling. Those same flaws have worked their way into consumer-facing AI software. In 2015, Google tweaked Photos to stop using a label after software engineer Jacky Alciné found the app was misidentifying his Black friends as “gorillas.” You can trace many of those problems back to the datasets organizations use to train their software, and that’s where an initiative like this can help. A recent MIT study of popular machine learning datasets found that around 3.4 percent of the data in those collections was either inaccurate or mislabeled.
While Facebook describes Casual Conversations as a “good, bold first step forward,” it admits the dataset isn’t perfect. To start, it only includes people from the United States. The company also didn’t ask participants to identify their origins, and when it came to gender, the only options they had were “male,” “female” and “other.” However, over the next year, it plans to make the dataset more inclusive.
(26)