The Penetration of Artificial Intelligence in Indian Railways
The Penetration of Artificial Intelligence in Indian Railways
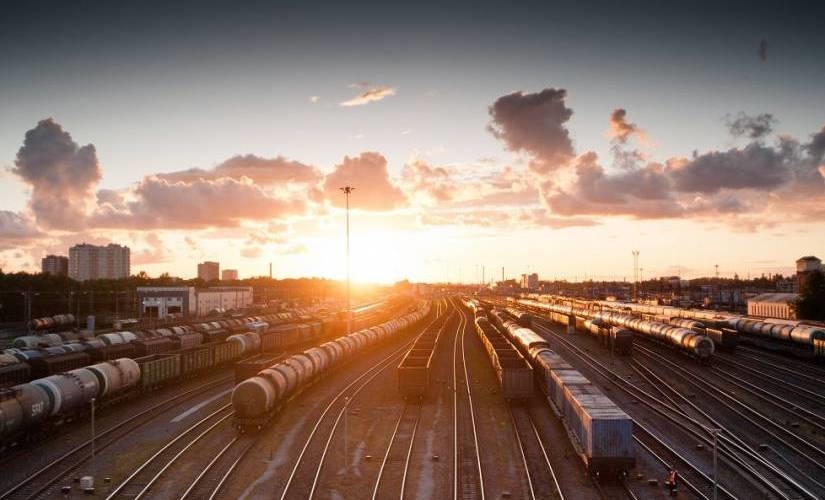
The advancement of railway technologies is meant to accommodate the growing population. Following the expectation coincides with the evolution of digitization. The popular use of online services through the internet allows the integration of everything to provide better services. Everything is computable and predictable by the processing of accumulated data. This leads everyone to think about the upcoming role of Artificial Intelligence in Indian Railways.
Artificial Intelligence in Indian Railways
Indian railways are the fourth largest network in the World with a track length of over 70,000 km in April 2019 and a network of more than 20,000 passenger trains. Managing all aspects – operation, maintenance, scheduling, repair or monitoring has always been a tough task for the Indian railways. The task is difficult due to the problem of integration of various systems such as signaling, telecom, operation, rolling stock, electrical, information technology, traffic, infrastructure, etc. and the involvement of human factors.
One way of trying to meet the demand is to enhance railway infrastructure. The infrastructure has been the key feature of current projects such as the Konkan Railway double-track expansion and the introduction of bullet train along the Mumbai-Ahmedabad High-Speed Corridor. But the question is why to overlook the increase in throughput to meet the increasing demand by the incorporation of Artificial Intelligence, machine learning, and self-control systems.
These systems are designed to improve the reliability of existing infrastructure and make up for the one-time heavy investment. The heavy investment can eliminate the need for human interference and provide the required level of safety and speed up-gradation. It should be possible to do more with the hardware if we can make the ‘software’ more efficient. Having more efficient software includes greater information sharing, lower latency, and smart algorithms. This is why many OEMs and startups are now investigating the feasibility of services based on Artificial Intelligence worldwide.
Feasibility of Artificial Intelligence in Indian Railways
We should analyze the fact if whether the amount of data required for the simulation and mathematical modeling is available. Let us consider various areas of interest separately for Indian railways.
- Operation – Artificial intelligence for any system requires a huge amount of linkable data. In Indian railways, the network already runs on the SCADA system, so a vast amount of operational data is available for modeling and training purposes.
- Infrastructure – Digitised versions of railway infrastructure should also be readily available. The same argument can be made for rake information and crew rosters.
- Tracks and Rolling stock – The data for predictive maintenance of the tracks or trains may not be available in India. But the physics of these problems does not vary with geography. Therefore, one can use data from other countries for training these models.
- Signal and Telecom – The history of events may be obtained from the data loggers of the interlocking system. This can help to schedule the movement of trains and also to manage machine-driven operations.
Although we have good availability of the data, the key challenge would be in developing a framework where researchers can start with several individual problems, and then integrate them effectively. Hierarchical or more generic architectures are effective in handling such intentions. These could be used from an algorithmic standpoint. The need is to make the various systems talk to each other effectively. This is where expertise in software/hardware architectures and system integration is required. A typical integration scenario is shown in Fig 1 below.
Applications of Artificial Intelligence in Indian Railways
There is a long-range service spectrum that AI can provide depending upon the level of efficiency and need. The feasibility of some of the primary requirements of Railways which Artificial Intelligence can provide is discussed below:
1) Train Scheduling
All signaling rules assume that tracks at stations are occupied by at most one train at a time. This can be ensured by algorithms, simulation models, graphs, heuristics and control systems with the required degree of Artificial Intelligence in Indian Railways.
The information which can be obtained from AI shall include:
(i) time duration from the ?rst event to the last event,
(ii) the total or average running time of trains,
(iii) the priority-weighted running time of trains,
(iv) robustness of the timetable to deviations, and combinations thereof.
This will make sure that
(i) either a track section between two stations is occupied by at most one train at a time (in absolute block signaling), or
(ii) every piece of track between two signals is occupied by at most one train at a time (in automatic block signaling).
Currently, railway networks do not use automated algorithms for this function. They instead rely on the training and experience of controllers (dispatchers) to take decisions. They cannot process a large amount of contextual information, Neither they can meet the demand for small turnaround time for decision making.
But the above-mentioned approach will generate high-level timetables and schedules of train movement. They will specify tracks to be occupied, the time required for switching tracks, and signaling requirements. And the parameters of immediate conflict can be evaluated in real-time. Hence, instant scheduling decisions can be taken, generating microscopic schedules.
With AI, one can develop iterative optimization approaches or graph-based models to compute low-level timetables with real-time decision support using heuristics as summed up in Fig 2 below.
Artificial Intelligence also offers a way of training algorithms to react to disturbances quickly and yet with near-optimal solutions.
Fig 2: A typical Scheduling Algorithm using AI
2) Controlling the speed profiles of trains
An AI-based con?ict resolution scheme shall not only achieve hard signaling (signal aspects) but more optimum approaches such as train speed management. Both the energy consumption of the train and total delay depend on the speed pro?le used between stations. Using Reinforcement Learning (RL) or dynamic programming, energy-efficient speed pro?les for single trains can be computed at the initial level. However, for broader applications in the country, the future possibility is the development of AI techniques that can:
(i) interact with human train operators without increasing their load,
(ii) be implemented by humans in the loop, and
(iii) detect obstacles on the tracks.
3) Delay Prediction and Reduction
One major role of Artificial Intelligence in Indian Railways can be the prediction of train delays. This is an important consideration for the highly limited nature of railway networks. Currently, there is no mechanism in IR to take corrective actions for the delay in train timings. Such delays are caused due to train priorities, downstream con?icts with other trains, freight loads, and irregular stopping times. A human cannot process all of these factors simultaneously, or come up with an optimal solution for the network as a whole.
Accurate delay predictions due to the incorporation of Artificial Intelligence in Indian Railways would help dispatchers (controllers) in downstream portions of the network. It would also improve the passenger experience by providing early updates regarding their journeys. A system to predict delay time would learn from past train delay data, predict how long each delay will be, and use a cloud-based service to deliver updates.
An AI-driven approach is a ‘sense-analyze-respond’ system to predict and correct delays. The ‘sense’ part of the program gathers data about the status of trains in the network. The ‘analyze’ part calculates the implications of each possible option. And the ‘respond’ part allots the computed track resources to each train based on physical capabilities and safety standards.
4) Asset management
The foolproof working of the signaling system is important for safe train operations. Railways completely depend on the health of its signaling assets along with real-time information. Most of the delays happen due to the failure of signals. So far, Indian systems follow a manual maintenance system and find-and-fix methods. But the adoption of Artificial Intelligence in Indian Railways can help predict failures by remote condition monitoring of the system well in advance.
This can be possible by embedding smart sensors on critical rail components and take necessary preventive actions. Inputs shall be collected on fixed intervals and sent to a central location (such as operations control center or OCC). As a result, any problems in the signaling system would be detected on a real-time basis.
This is depicted in Fig 3 below. Trains equipped with smart sensors and GPS transmit component-wise health status and location to the AIC (Asset Intelligence Center). The AIC which maintains the digital database of all railway assets also collects inputs from the safe distance warning (SDW) system embedded in the track-side cameras about the train/wagon defects and electronic damage notifications (also transmitted to the driver).
Once such information is gathered and integrated, data analytics in the form of RAM / LCC (Reliability, Availability, Maintainability and Lifecycle Cost) diagrams are generated which calculates the cost overhead in maintaining the particular train component at a given time and conditions and also generates a digital Rulebook (say, Rulebook 4.0) which shall provide an easy access to the maintenance policies in the form of a structured data model for use by concerned operators and workshop staff in the future.
The AI-recommended decisions based on dynamic algorithms and policies as per the digital Rulebook are then encapsulated into a consolidated maintenance schedule inside the Digital Fleet Control Module and is compared against the workshop capabilities to generate demand for material and labor. This demand is eventually fetched to the workshop Digital Interface in the form of digital order.
As of now, remote monitoring of signaling is operational in Britain. The system involves data transfer through a wireless medium (3G, 4G, and high-speed mobile). The data based on these inputs are utilized with the help of AI for prescriptive Big Data analytics. This allows the detection of signaling asset defects, automated self-correction (through machine learning) and informed decisions.
Indian railways have decided that trial be taken up in two sections of Western Railway and South Western Railway at Ahmedabad-Vadodara and Bengaluru-Mysuru.
5) Intelligent Signalling Systems
These can be built by generating a ‘Digital twin’ of a railway section. A digital twin is a simulation model of a real physical system and its operations. multiple data sources continuously update the virtual replica and change its state to represent the effects on the physical counterpart.
A helicopter or a drone equipped with laser scanners and cameras can capture images of the whole section and simulate a fully mapped-out, three-dimensional digital model. Then a digital guide of all assets can be developed. This can include thousands of kilometers of track, signals, points, telecom towers, stations, cable ducts, bridges, tunnels, and level crossings.
The benefits of such a model include:
-
Automation of design
‘Digital twin’ can help semi-automate the design with total precision, saving months of work. It eliminates the need to go onto the site and manually measure and estimate gradients, determine equipment requirements such as cable and connectors, locate infringements and track crossings.
A ‘digital twin’ has been developed by Alstom for the busy UK’s rail network along the West Coast Main Line (WCML) for the Pendolino train. The entire train fleet encompasses fifty-six trainsets along with five maintenance depots. The maintenance of such a complicated system with the required degree of prediction needs many aspects for consideration which would not happen without a digital twin.
-
Scheduling
Daily operating requirements for the routes and timetables regarding the trains and capacities can be estimated.
-
Maintenance
Frequency and parameters (such as time or mileage) for train inspection as well as the details of preventive and corrective maintenance for the depots can be determined.
-
Asset safety and reliability
The digital model will indicate the instance of theft or the possible failure of any signaling gear. This ‘predict-and-prevent’ model will save money, increase capacity, and eliminate any unplanned delay or service cancellation. The added cyber-security option can be utilized to deliver a secure online key management system for the trains to communicate securely with the wayside signaling system using encryption keys.
As shown in Fig 4 above, data from track and train is collected by smart sensors and cameras which acts as an input for digital twin modeling. Hence two parallel layers – Physical and Digital are created on the network which communicates with each other through suitable interfaces.
The digital model calculates the operation time and interval of the train which may be applied to the AI-based risk evaluation algorithms. The Real/Physical layer monitors and adapts to the changes in rules and regulations by the government and then sends the information to the digital layer which updates risk calculations whenever new information is received.
Finally, on the basis of the collected information, the operation plans so generated are tested on the digital model and proposed to the physical layer for encapsulation.
6) Ensuring public safety
The advisory roles in train positioning, acceleration, safe braking, and interlocking can eliminate the need for direct control of the driver. The first autonomous tram launched by Seimens in Potsdam, Germany in 2018 can avoid the threat of people walking in front of a moving vehicle.
With the help of sensors, lasers, and cameras, any obstacle on the path can be detected. This helps in taking the split-second decisions bringing AI-assisted tram to an abrupt stop. It is still in the development phase but it surely has a great deal to offer the wider autonomous vehicle market that is speeding up at a high rate.
To understand this approach, let us assume an Operation Control Centre as shown in Fig 5 above which contains the central server for traffic monitoring and management of the entire railway section. The radio balize fitted on the track transmits the location and signal aspects to the train through on-board sensors and antenna.
This information along with the train’s health status is sent through an AI-based train monitoring module to the communication module. This module also collects track-based information such as track failure or an obstacle on the route from the trackside processor and sends it to the OCC.
The OCC on the other hand also receives the risk analysis output from the probabilistic AI modules (as explained before) and feeds the cumulative information to the deterministic AI algorithms to generate a final decision. The outcome is conveyed to the train driver’s screen and the result is an automatic instantaneous response in the form of acceleration, braking, closing or opening of doors, etc.
Other Applications
1) Predictive maintenance.
Smart detectors can be fitted in axle counters, track circuits, point machines, signals, and rolling stock so that any flaws can be detected. They can measure the peak load exerted by railway carriages as they pass over the tracks. As a function of time and location, the historical data is available in the form of track geometry and fluctuations underneath the carriages.
The data is translated into defect measurements. Hence any developing faults are sensed well in advance. The remaining life of tracks and wheels is predicted almost accurately. If the modeling is good enough, maintenance can be carried out (automatically in case of AR) before the faults become critical but not so frequently that the cost becomes prohibitive.
Predictive maintenance hence enables the operators to quickly react to the existing issues and failures. It also detects any potential failures before they happen. Hence the need for lengthy root cause identification is eliminated. This leads to reduced maintenance costs, less (or zero) failure rate, quick repair and increased customer satisfaction.
2) Crew management.
Using linear programming and heuristics, an AI-driven adaptive approach can ensure that the operating personnel in a given railway section can adhere to the timetable or schedule by
1) Crew rostering – long-term distribution of personnel at various nodes of the network and
2) Crew scheduling – a short-term planning problem.
The rules that apply to both can be complex combinations of capacity, crew preferences, rest periods, overnight stays, and other factors.
3) Data Management.
The rail industry, like any public system, uses many procedures. Such applications generate a vast amount of data that will only grow in the future and hence difficult to handle. Under such a scenario, cloud-based AI technology can allow railways to store the collected data digitally, which will save costs and provide consistent accessibility.
Another merit of Cloud technology is that it can easily be integrated and connected to other software. So operators can immediately have organized information for fast decision making.
4) Biometrics.
Biometric ticketing includes retina scans, voice verification, vein scans, facial recognition, and fingerprint scans. Infrared light-based cameras capture the length, texture, and shape of body parts in immense detail. The data shall be used for ticketing and registration purposes. This would greatly improve the overcrowding of passengers at train stations and eliminate the need for ticket counters.
Currently, companies like Customer Clever and Bristol Robotics Laboratory of the UK are already developing this system. It cannot be tricked by photographs, can distinguish between identical twins, and can even recognize people wearing glasses. The system can also already identify people while they are walking. This would allow stations to completely replace ticket gates.
Challenges
Artificial Intelligence seems to be a powerful cutting-edge solution for almost all the areas of railway systems mentioned above. But the technology has a range of challenges to be faced during the planning stage:
1) Easy penetration.
The techniques developed must be usable across all problem instances, without extensive retraining. A scheduling algorithm developed for one portion of the railway network must be usable in any other portion.
2) Variability.
Not every problem instance (for example, a piece of the railway track) contains the same number of inputs and decisions. Many algorithms under the AI umbrella (for example, deep learning) handle only a ?xed input-output size. Careful design can meet the discrepancy between raw methodology and domain constraints. But this requires time and e?ort.
3) Need for detailing.
The black box approaches are acceptable for conceptual studies and testing. But they are not viable for safety-critical applications of the railway. Instead, machine learning approaches shall be required in the form of decision trees or neural networks with small input-output sizes.
4) Adherence to operating rules, procedures, and constraints.
Real-world systems and national rules need to source the data requirements for building any methodologies. They must allow for AI-based measurements and prevent errors in those calculations. The need shall be to meet the integration and connectivity requirements within the allowed limits of transmission latency.
5) Interoperability.
With large sharing of data shall come the need for closer collaboration. This includes operations, communications, and integration of data amongst different OEMs. Hence different AI-based solutions need to integrate into a cohesive framework.
6) Cost and complexity.
The modification of existing systems to IT-based subsystems shall require thoughtful planning and a huge cost.
Conclusion
The digital railway is a developing reality worldwide. It commits to providing advanced technology for both trains and track to deliver faster, more frequent services for passengers and businesses alike. Our economy can have a massive boost by the involvement of artificial intelligence in Indian Railways.
But this can take a few decades to follow since AI-based train systems are currently under the testing or development stage. Also, the overhead in terms of cost, complexity and the high level of technical competence cannot be ignored.
The post The Penetration of Artificial Intelligence in Indian Railways appeared first on ReadWrite.
(98)